How to Use
There are no special requirements for the tool, such as software or specialist involvement. The tool is especially helpful for project teams and the project managers of promising (BwN) initiatives and can be used in the setting of a regular project meeting. The tool mainly consists of three steps:
- Uncertainty identification
- Uncertainty classification
- Uncertainty management
1. Uncertainty identification
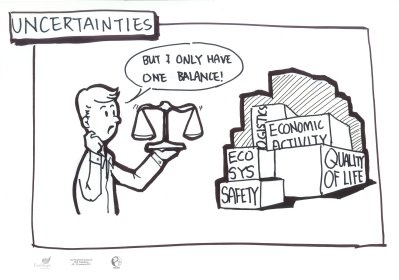
When identifying the most important uncertainties in a BwN project’s development process, actors need to focus on uncertainties that can hamper development, cause budget overruns or cuts, influence milestone decisions or even cause the cancellation of the entire project. The number of uncertainties in a project using BwN design principles is by definition very high, as this is an inherent characteristic of this type of projects. Therefore, do not focus on the numerous “minor” issues, but concentrate efforts on the (few) uncertainties that can become a major concern.
Note that there is a difference between true uncertainty and perceived uncertainty. Some issues are “truly” uncertain, for instance because there is insufficient knowledge about a subject. Some other issues can be perceived to be uncertain whereas they are not. For instance, some stakeholders may expect the project to have a specific negative effect, while it is generally known among experts that this effect is highly improbable. Yet, perceived as well as true uncertainties can influence the project´s development and are therefore equally relevant. The role of perception or framing in uncertainty studies is discussed in more detail below.
2. Uncertainty classification
Uncertainty is defined as the situation in which there is no unique and complete understanding of the system at hand (Brugnach et al., 2008). Following this definition, we distinguish three types of uncertainty:
- Unpredictability – uncertainty due to unpredictable or chaotic behaviour of e.g. natural processes, human beings or social processes (Walker et al., 2003; van Asselt and Rotmans, 2002). Weather conditions are a typical example of an uncertainty that is unpredictable. The uncertainty is not reducible by doing more research, as the weather is variable and unpredictable. Lorenz (1963, 1969) already showed that an indicative prediction of the weather cannot be given more than 2 weeks in advance, as minor changes in initial values can have a major impact on the evolution of the system´s state;
- Incomplete knowledge – uncertainty due to the imperfection of our knowledge, e.g. due to lack of specific knowledge, data imprecision or approximations (Walker et al., 2003). For example, in the case of the Sand Engine, the uncertainty about the effect of the sand nourishment on groundwater levels is due to a lack of knowledge. This uncertainty is reducible by additional research;
- Multiple knowledge frames – uncertainty due to the presence of multiple knowledge frames or different but (equally) valid interpretations of the same phenomenon, problem or situation (Brugnach et al., 2008, 2011; Dewulf et al., 2005; Kwakkel et al., 2010). For example, Brugnach et al. (2008) studied an irrigation problem in a Spanish river basin. While farmers framed the problem as a situation of water shortage, the government framed it as a situation of overconsumption of water resources. Both interpretations of the problem can be valid, but lead to different action paths and solutions. Hence, the presence of multiple knowledge frames causes uncertainty in decision-making.
It is important to distinguish these types of uncertainty, as different strategies are needed to manage each of them (Brugnach et al., 2008).
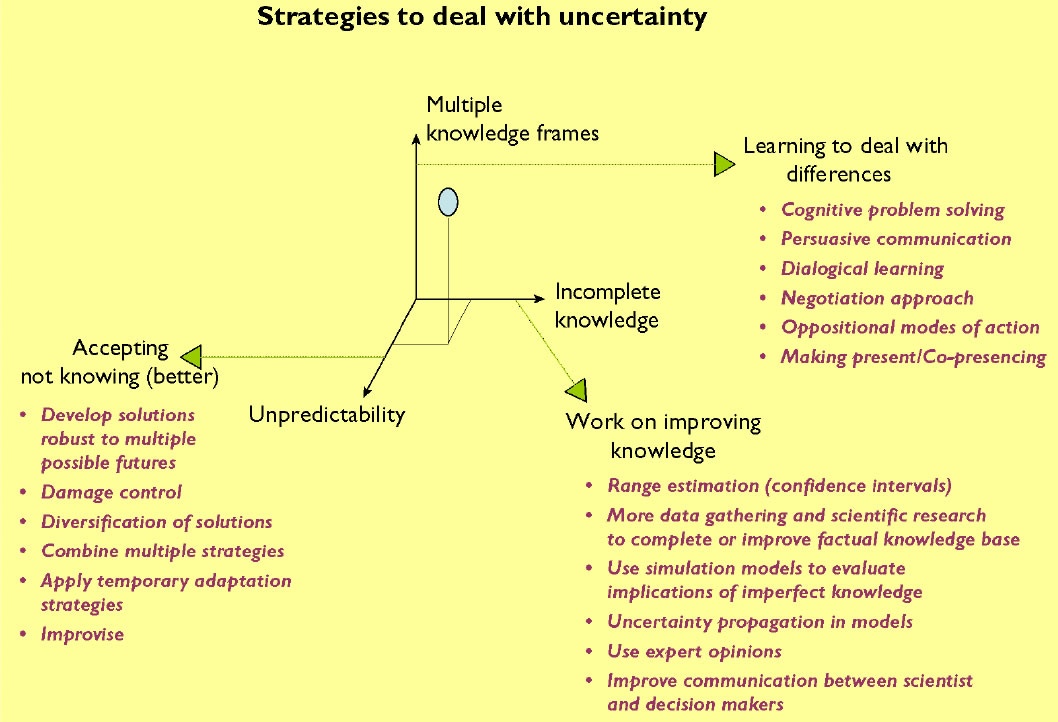
3. Uncertainty management
Managing uncertainty is of major importance in EDD. Brugnach et al. (2008, 2009) give a clear overview of strategies that can be applied to manage each of the three types mentioned above(see Figure as well):
- Unpredictability – The appropriate strategy here is to accept that we cannot know better. An unpredictability CANNOT be reduced by doing more research due to its inherently chaotic and variable behaviour;
- Incomplete knowledge – Here the strategy should be to increase or improve the available knowledge. In principle, this uncertainty can be reduced by knowing more or better. Yet, the opposite can also be true: more information may also enhance our awareness of knowledge gaps, and thus increase uncertainty (Van Asselt, 2000);
- Multiple knowledge frames – Dealing with framing differences is the strategy to be adopted here. If multiple frames are present, additional knowledge will not solve the problem. Seeking mutual understanding and shared commitment is the way to solve the problem of ambiguity.
The figure is a graphic representation of these strategies. The figure was taken from a report by Brugnach et al. (2009) from the NeWater project. It specifically addresses uncertainty in a similar context as BwN, namely ‘adaptive water management’.
4. Advice and recommendations
The BwN project ‘Coping with uncertainty’ yielded several results regarding uncertainty using the method described above. The outcomes of this analysis provide important lessons where to expect uncertainty in future BwN projects and how to deal with it. These lessons are briefly discussed below.